How to support the driver of conditionally automated vehicle in takeover situations using machine learning
Ingénierie et Architecture
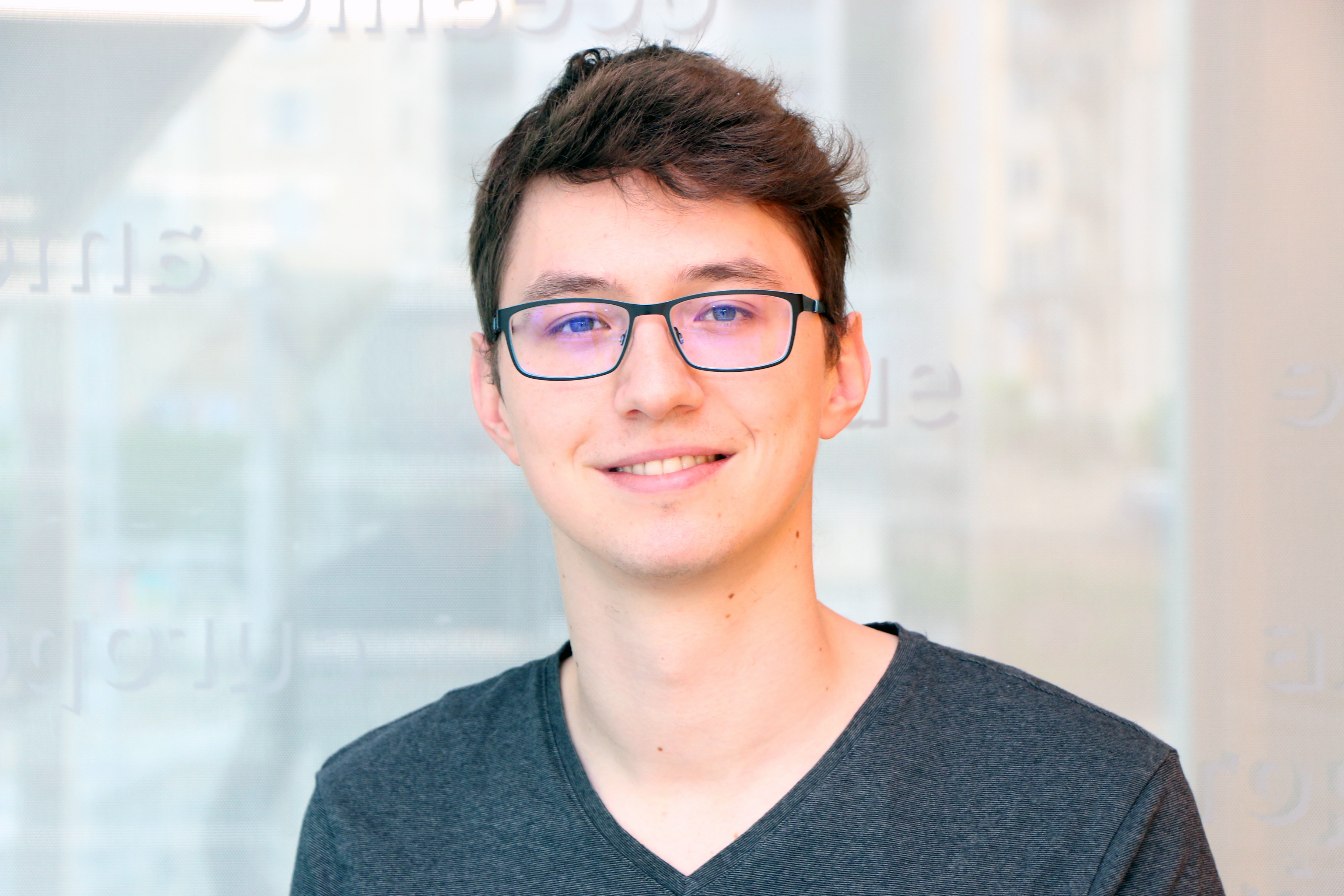
Conditionally automated vehicles are becoming increasingly common on the roads, as the technology allowing them to drive without human intervention improved drastically these last few years. Before we can move on to fully autonomous vehicles that are completely independent of a human driver, more and more advanced semi-autonomous models are being developed. Nonetheless, problems specific to this conditionally automated status have emerged, such as the necessity for a driver, who is most of the time out of the driving loop, to intervene in specific scenarios. These handovers of control, or takeover, being short and critical situations, can potentially lead to accidents if executed poorly or too late by the driver. The analysis of the driver performance in a takeover situation allows us to say if the process was executed correctly, but it can only be done after the takeover is resolved. In addition, there are numerous was to convey a takeover request to the driver, essentially by choosing the modalities used to communicate it to the driver (auditory alert, icon on the dashboard, etc.). The modality choice is critical since it influences the reaction of the driver. This research paper offers methods which can be used to improve driver performance in takeover situations, specifically in the form of a smart agent. This agent is based on Machine Learning algorithms to optimize the Human-Machine interaction between the driver and the car requesting a takeover to be done. In concrete terms, the agent is able to choose the modalities of the takeover request. To do so, physiological signals of the driver, their secondary activity and the external environment are monitored and analyzed in real-time. This allows the agent to predict the driver’s performance if a takeover is about to occur. It can then evaluate the impact of the takeover request modalities on the takeover performance. Using this information, the agent is able to propose the optimal interaction with the driver, at any given time, allowing them to respond to a takeover request to the best of their capacity. The evaluation of the agent has shown its potential as a tool to increase the safety of the driver and their environment in case of takeover situations. The highlights of the factors impacting the takeover performance, the smart agent itself and the discussions around the methods proposed in this paper should allow researchers in the field of autonomous vehicles to improve the safety of their conditionally automated systems in the future.